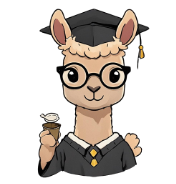
Cream of the Crop
Harvesting Rich, Scalable and Transferable Multi-Modal Data for Instruction Fine-Tuning
Abstract
Our Method
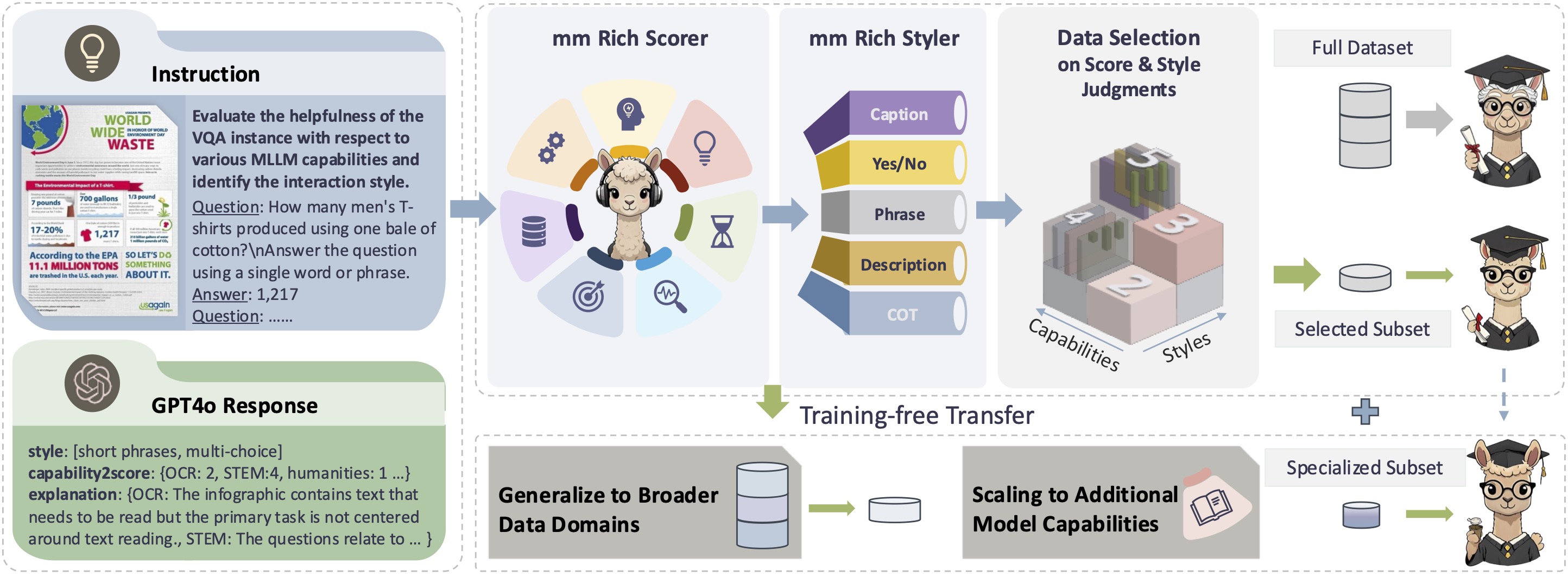
(L) We assess GPT-4o's evaluations of rich capabilities on a scale from 0 to 5, while meantime prompting the identification of the user-model interaction style.
(R-top) The small amount of derived sample-scores-style triplets is employed to instruct the pretrained task model to rich capability scorers and styler, ie our mmSSR.
It facilitates the analysis and sampling of candidate data points at the scale of millions, ensuring a subset that is both high-quality and diverse, while maintaining minimal time and resource expenditure.
(R-down) The fine-grained mmSSR can also directly generalizes to other data domains, and support efficient scaling in data quantity and capabilities.
Experiments
Baseline Comparison
MMBenchen-v1.1 | MMStar | MMMU | MMVet | BLINK | MMT-Bench | MME | AI2D | ScienceQA | MathVistaMINI | >Rand | /FULL | |
---|---|---|---|---|---|---|---|---|---|---|---|---|
5% Budget | ||||||||||||
Random | 73.74 | 47.98 | 43.70 | 42.34 | 50.61 | 58.87 | 2004.50 | 73.07 | 81.52 | 45.47 | - | 89.29 |
PPL-mid | 67.34 | 45.27 | 38.98 | 30.18 | 45.27 | 54.33 | 1887.71 | 66.74 | 74.76 | 31.40 | 0/10 | 78.31 |
PPL-si | 71.98 | 44.67 | 38.48 | 35.14 | 54.10 | 57.98 | 1856.79 | 67.84 | 78.24 | 36.50 | 1/10 | 83.10 |
Deita | 72.91 | 47.47 | 41.28 | 40.23 | 52.59 | 56.57 | 1956.50 | 70.76 | 79.57 | 36.10 | 1/10 | 85.79 |
CLIP | 74.23 | 47.27 | 40.08 | 35.73 | 52.96 | 56.73 | 1902.65 | 73.61 | 78.63 | 39.80 | 3/10 | 85.41 |
E5-V | 70.90 | 43.00 | 38.78 | 38.44 | 49.94 | 54.65 | 1810.47 | 66.58 | 77.54 | 37.40 | 0/10 | 81.87 |
COINCIDE | 72.76 | 48.33 | 43.17 | 45.60 | 49.43 | 57.50 | 1852.66 | 73.15 | 79.62 | 45.40 | 3/10 | 88.47 |
mmSSR | 77.79 | 53.33 | 43.27 | 43.53 | 51.83 | 59.16 | 1938.68 | 77.66 | 88.45 | 52.00 | 8/10 | 93.20 |
10% Budget | ||||||||||||
Random | 74.57 | 51.57 | 44.72 | 42.91 | 52.59 | 58.99 | 2033.28 | 74.42 | 84.33 | 47.80 | 0/10 | 91.70 |
PPL-mid | 63.54 | 46.87 | 39.08 | 36.93 | 45.90 | 54.30 | 1831.03 | 67.23 | 73.87 | 39.50 | 0/10 | 80.72 |
PPL-si | 74.69 | 49.80 | 41.28 | 40.60 | 53.09 | 57.95 | 1841.11 | 75.16 | 80.71 | 40.40 | 3/10 | 87.63 |
Deita | 75.39 | 48.80 | 43.77 | 42.25 | 54.48 | 57.40 | 1996.34 | 71.60 | 78.33 | 40.80 | 2/10 | 88.72 |
CLIP | 75.23 | 49.87 | 40.38 | 37.16 | 53.59 | 59.35 | 1921.04 | 76.62 | 80.07 | 41.00 | 4/10 | 87.69 |
E5-V | 70.51 | 45.13 | 38.78 | 39.59 | 50.57 | 55.10 | 1787.94 | 68.94 | 77.54 | 37.20 | 0/10 | 82.76 |
COINCIDE | 75.23 | 49.73 | 44.77 | 42.52 | 50.69 | 58.71 | 2027.58 | 74.77 | 82.05 | 47.00 | 3/10 | 90.66 |
mmSSR | 77.32 | 53.27 | 45.06 | 42.98 | 54.10 | 59.61 | 2045.00 | 78.76 | 89.94 | 52.40 | 10/10 | 94.75 |
30% Budget | ||||||||||||
Random | 78.25 | 54.60 | 44.40 | 46.10 | 55.23 | 59.61 | 2092.60 | 78.28 | 88.32 | 52.57 | - | 95.82 |
PPL-mid | 73.99 | 54.93 | 43.97 | 41.01 | 53.09 | 58.78 | 2036.54 | 77.20 | 87.01 | 56.40 | 2/10 | 93.77 |
PPL-si | 72.52 | 48.33 | 42.57 | 43.62 | 51.83 | 55.07 | 1976.46 | 76.55 | 78.48 | 42.20 | 0/10 | 88.22 |
Deita | 76.93 | 54.13 | 43.67 | 44.04 | 55.11 | 59.66 | 2042.63 | 79.50 | 83.54 | 50.30 | 2/10 | 94.05 |
CLIP | 74.30 | 53.80 | 43.07 | 45.87 | 51.95 | 59.16 | 2039.14 | 80.02 | 83.99 | 48.80 | 1/10 | 93.07 |
E5-V | 74.30 | 46.07 | 43.27 | 47.80 | 50.32 | 57.85 | 1955.13 | 74.45 | 81.61 | 43.70 | 1/10 | 89.52 |
COINCIDE | 78.02 | 55.47 | 45.66 | 46.24 | 52.84 | 59.80 | 2047.37 | 79.73 | 84.33 | 55.10 | 6/10 | 95.82 |
mmSSR | 79.57 | 57.53 | 44.87 | 48.49 | 56.24 | 59.83 | 2132.93 | 81.25 | 92.46 | 57.40 | 10/10 | 99.11 |
FULL | ||||||||||||
LLaVAOVSI | 80.57 | 59.40 | 45.16 | 47.16 | 56.87 | 60.73 | 2117.56 | 81.87 | 92.76 | 59.60 | - | 100 |
Why our mmSSR for Scoring and Styling?
Why rich capabilities over the straight-forward quality metric? The comparison between mmSSP(oor) and mmSSR(ich) (proposed) demonstrates that the latter is more effective in capturing the richness and diversity of multi-modal data, which is crucial for the selection.
Can we directly adopt the task model for rich scoring and styling? Not recommended. The comparison between mmSSR + LLaVA-OVSI (finetuned) and mmSSR(ich) (proposed) shows that the open-source model is not as effective as proprietary models (ie, GPT4o we used) in the instruction following of the scoring and styling tasks.
Can we use open multi-modal models for rich scoring and styling? Acceptable when the time/computation/API budget is very constrained. Note that the performance of this approach still lags noticeably behind the specialized models we have developed from state-of-the-art proprietary model, as indicated by mmSSR + Qwen2-VL vs mmSSR(ich).
MMBench-en-v1.1 | MMStar | MMMU | MMVet | BLINK | MMT-Bench | MME | AI2D | ScienceQA | MathVista-MINI | >Rand | /FULL | |
---|---|---|---|---|---|---|---|---|---|---|---|---|
5% Budget | ||||||||||||
Random | 73.74 | 47.98 | 43.70 | 42.34 | 50.61 | 58.87 | 2004.50 | 73.07 | 81.52 | 45.47 | - | 89.29 |
mmSSP(oor) | 75.85 | 51.27 | 42.97 | 44.27 | 51.95 | 58.14 | 1940.27 | 73.61 | 81.46 | 45.00 | 5/10 | 90.14 |
mmSSR + LLaVAOVSI | 77.40 | 50.60 | 44.77 | 41.10 | 54.35 | 58.62 | 1952.97 | 75.81 | 87.75 | 40.40 | 6/10 | 90.68 |
mmSSR + Qwen2-VL | 75.08 | 51.00 | 45.16 | 42.57 | 52.71 | 57.37 | 1955.78 | 74.74 | 84.88 | 48.90 | 8/10 | 91.37 |
mmSSR(ich) | 77.79 | 53.33 | 43.27 | 43.53 | 51.83 | 59.16 | 1938.68 | 77.66 | 88.45 | 52.00 | 8/10 | 93.20 |
10% Budget | ||||||||||||
Random | 74.57 | 51.57 | 44.72 | 42.91 | 52.59 | 58.99 | 2033.28 | 74.42 | 84.33 | 47.80 | - | 91.70 |
mmSSP(oor) | 77.24 | 50.40 | 44.27 | 42.52 | 53.47 | 59.48 | 2084.39 | 76.07 | 81.36 | 46.10 | 5/10 | 91.73 |
mmSSR + LLaVAOVSI | 77.79 | 54.40 | 44.67 | 42.02 | 54.98 | 58.23 | 2013.74 | 78.85 | 89.59 | 42.00 | 5/10 | 92.72 |
mmSSR + Qwen2-VL | 76.24 | 53.33 | 44.87 | 45.60 | 55.11 | 59.16 | 2012.94 | 76.75 | 87.11 | 52.70 | 9/10 | 94.59 |
mmSSR(ich) | 77.32 | 53.27 | 45.06 | 42.98 | 54.10 | 59.61 | 2045.00 | 78.76 | 89.94 | 52.40 | 10/10 | 94.75 |
30% Budget | ||||||||||||
Random | 78.25 | 54.60 | 44.40 | 46.10 | 55.23 | 59.61 | 2092.60 | 78.28 | 88.32 | 52.57 | - | 95.82 |
mmSSP(oor) | 77.86 | 53.13 | 45.76 | 48.03 | 54.85 | 58.78 | 2050.69 | 78.92 | 86.91 | 55.80 | 4/10 | 96.31 |
mmSSR + LLaVAOVSI | 77.55 | 54.53 | 43.37 | 44.72 | 55.23 | 58.59 | 1980.48 | 81.02 | 91.87 | 49.60 | 2/10 | 94.73 |
mmSSR + Qwen2-VL | 78.02 | 57.13 | 43.07 | 47.39 | 55.49 | 60.89 | 2096.60 | 81.64 | 90.28 | 57.40 | 8/10 | 97.91 |
mmSSR(ich) | 79.57 | 57.53 | 44.87 | 48.49 | 56.24 | 59.83 | 2132.93 | 81.25 | 92.46 | 57.40 | 10/10 | 99.11 |
FULL | ||||||||||||
LLaVAOVSI | 80.57 | 59.40 | 45.16 | 47.16 | 56.87 | 60.73 | 2117.56 | 81.87 | 92.76 | 59.60 | - | 100 |
Scalability in Data Budget
mmSSR in Cold, Warm and Hot Settings
Data selection methods, from CNNs to LLMs, are often suseptible to sensitivity to settings, such as benchmarks, target dataset, data volume and model arch etc.
Following the main experiments, we additionally validate mmSSR under varying data budget: colder (1%) and hot (40%, 50%) scenarios, achieving consistently superior performance when scaling the data budget volume.
Scalability in Capability
mmSSR for Specialized Capability Acquisition
We consider a data expansion scenario commonly encountered in real-world applications, scaling up the capability dimension within the existing data pool.
The OCR-favored samples newly acquired by our pipeline lead to steady improvements in specialized benchmarks, and they also contribute to the growth of general benchmarks or sustain their advantageous positions.
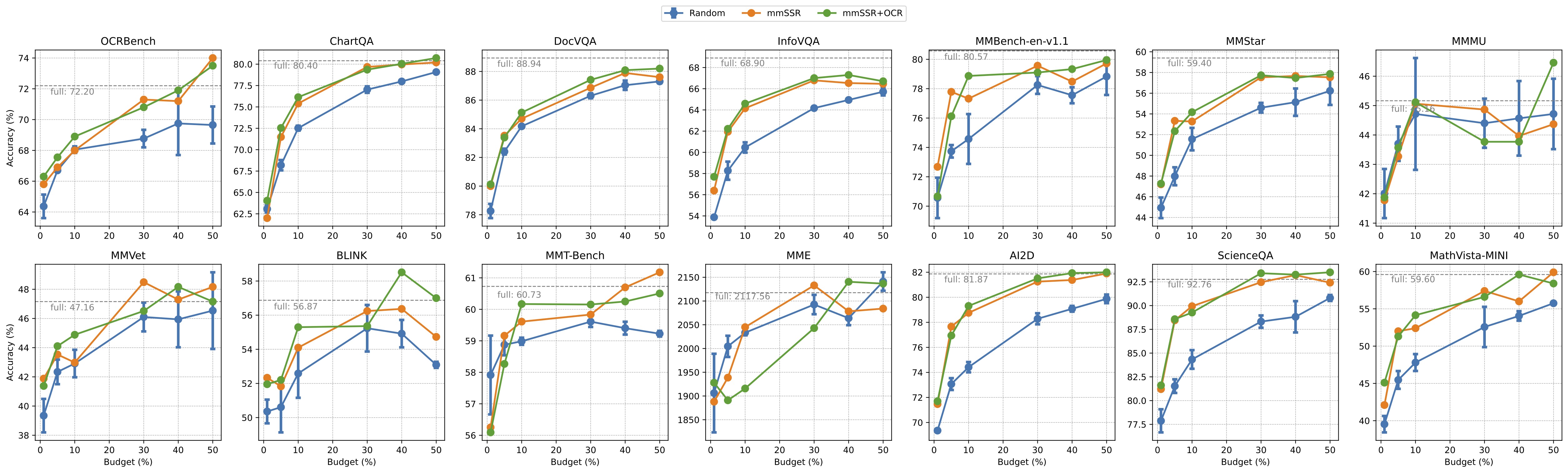
Model Transfer to Larger Data Pool
You might want to expand your data pool by adding new subdomains or sources to an existing dataset. This scenario plays out by first leveraging a subset of LLaVA-665K (12 sources) to train mmSSR and then generalize them directly to LLaVA-OVSI (90+ sources) for both inference and sampling. The models exhibit robust generalization capability to the larger data pool with open sources and novel knowledge.
Data Transfer to Different Model Arch
We also expect the selected subset to be generally applicable, instead of being dependent on specific architecture or data pool.
To verify the effectiveness of the subset selected by mmSSR that are finetuned from LLaVA-OVSI-7B, we use it to train a 0.5B model. Results show that its superiority remains, demonstrating strong robustness.
The Reasons Behind the Strong Generalizability of mmSSR
- Scores and styles are more generalizable than model responses: While model-based methods rely on their specific model responses (e.g., perplexity and embeddings) for data valuation, our mmSSR is instructed to score and identify instructional styles characterized by general semantics.
- Rich scores and styles are more generalizable than coarse-grained quality-like descriptors: For pretrained MLLMs to be finetuned, while the understanding of quality might shift, the intrinsic knowledge of fundamental capabilities and styles is more readily shared and transferable.
Analysis
Source distribution of LLaVA-OVSI vs 10% mmSSR selection
Source distribution of 10% mmSSR selection
Style distribution of 10% mmSSR selection
Score distribution of 10% (1-2) and 30% (3-4) mmSSR selection
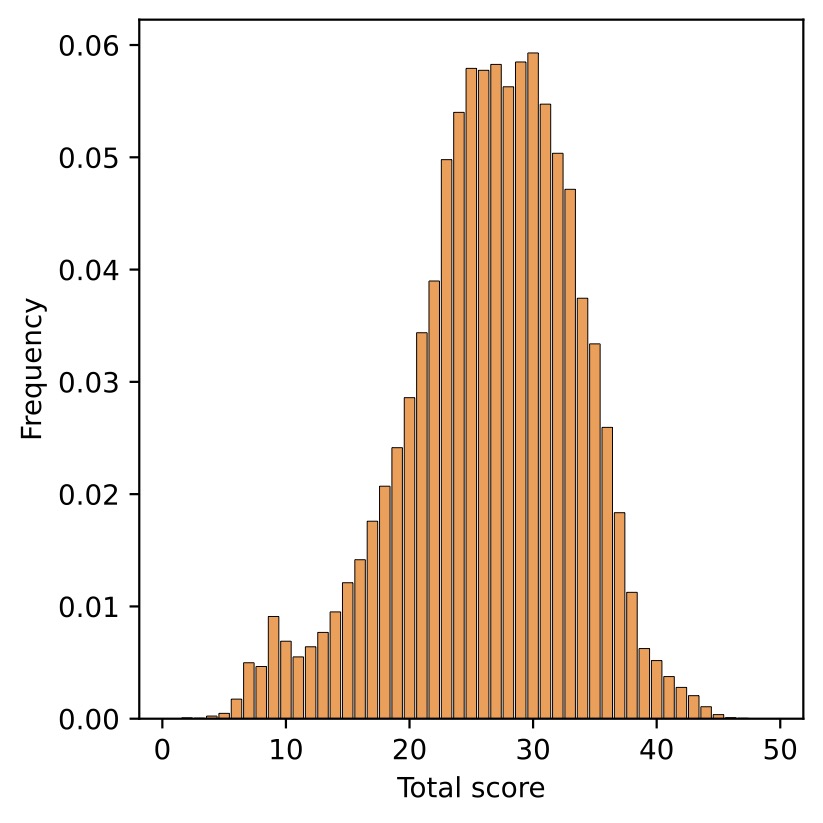
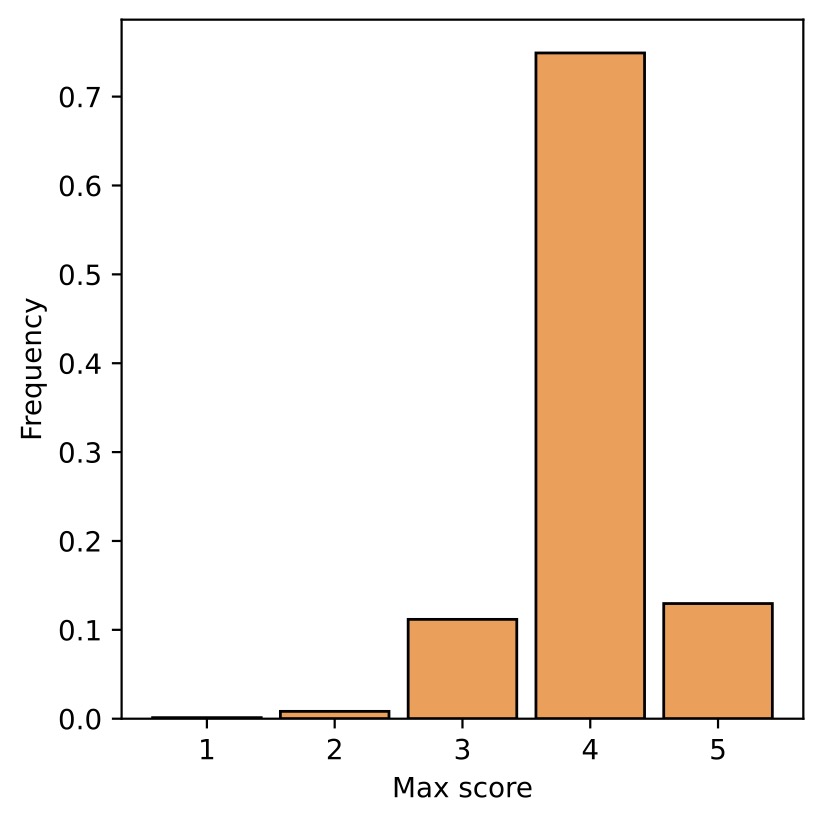
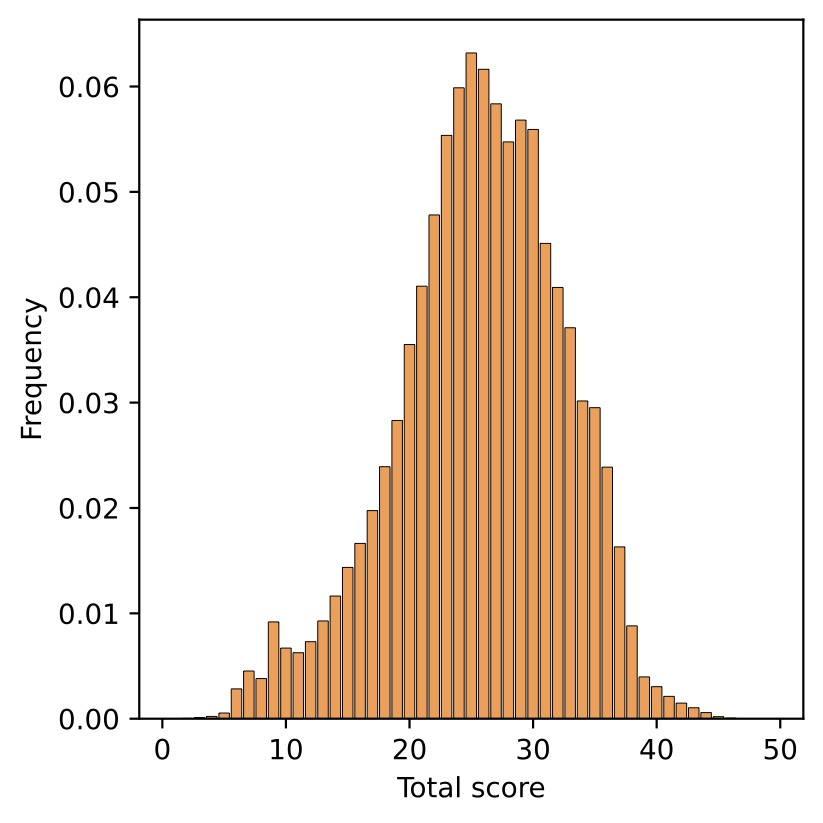
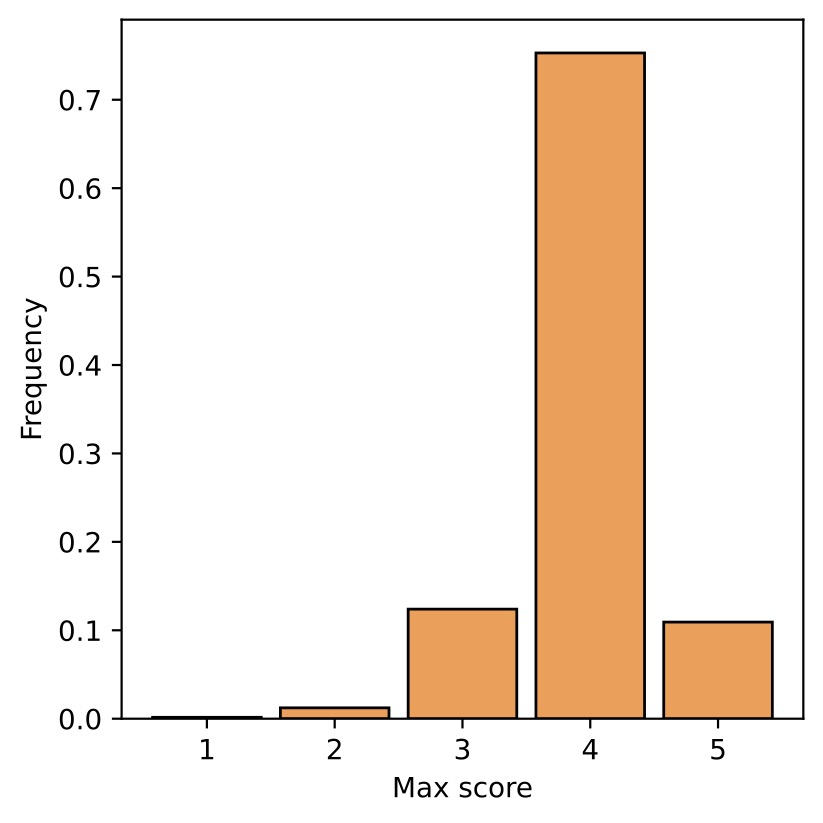
BibTeX
@article{lyu2025mmssr,
title={Cream of the Crop: Harvesting Rich, Scalable and Transferable Multi-Modal Data for Instruction Fine-Tuning},
author={Mengyao Lyu, Yan Li, Huasong Zhong, Wenhao Yang, Hui Chen, Jungong Han, Guiguang Ding, Zhenheng Yang},
journal={arXiv preprint arXiv:2503.13383},
year={2025}
}